Enhancing Machine Learning with Image Annotation
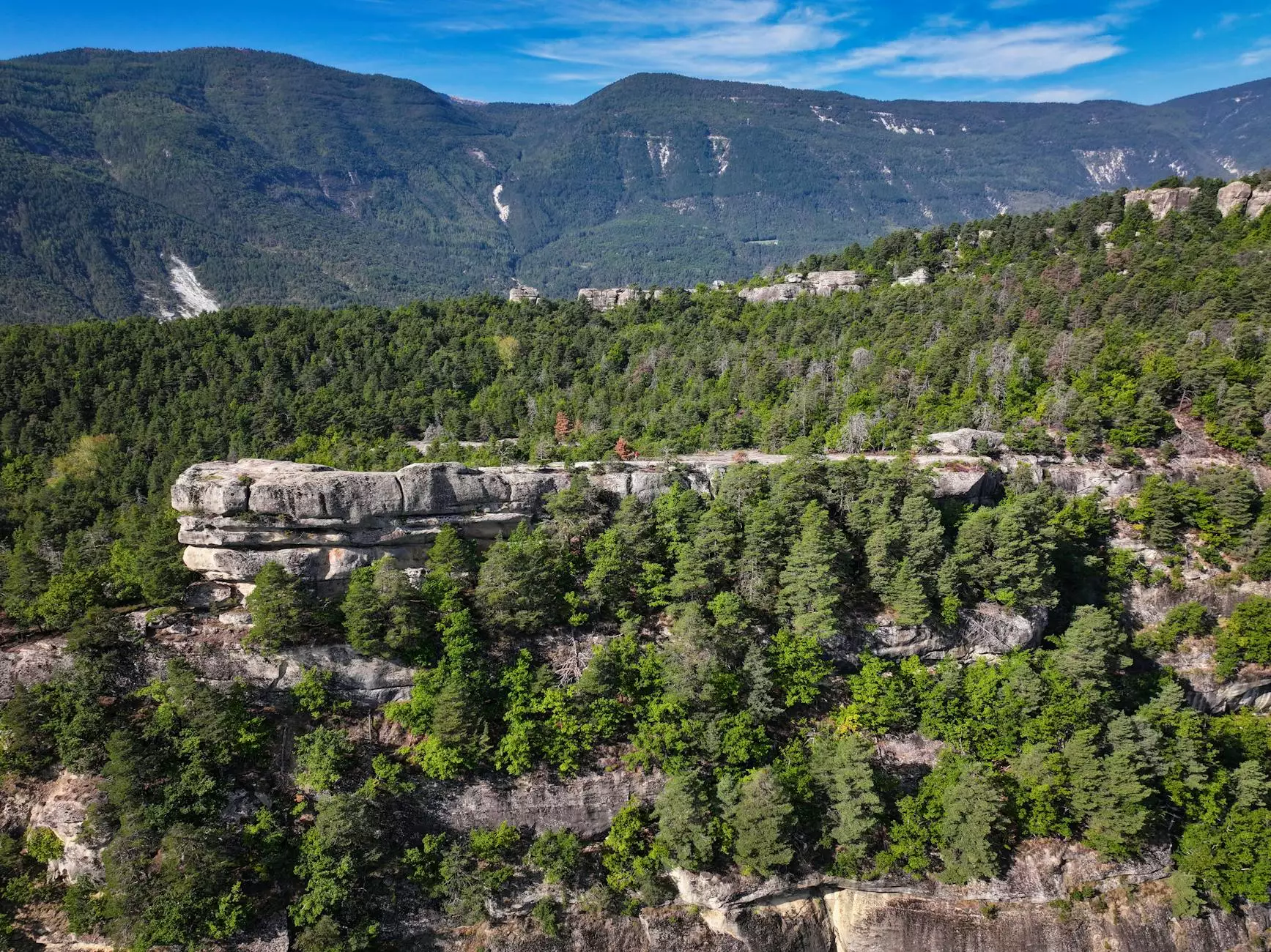
In the rapidly evolving field of artificial intelligence (AI) and machine learning (ML), data plays a crucial role in shaping the accuracy and efficacy of algorithms. One of the cornerstones of building reliable machine learning models is image annotation. This comprehensive approach not only improves model performance but also elevates the prediction capabilities of systems across various applications. In this article, we explore the ins and outs of image annotation for machine learning, particularly how platforms like keylabs.ai are transforming the landscape.
What is Image Annotation?
Image annotation is the process of labeling images with relevant tags or metadata to assist in teaching machine learning models. This can include the identification of objects, boundaries, and attributes within an image. By converting raw images into a structured dataset, image annotation allows algorithms to learn from the visual data presented to them.
The Importance of Image Annotation in Machine Learning
Machine learning depends heavily on data quality. The labels assigned during image annotation serve as the ground truth for machine learning models. Here are several key reasons why image annotation is essential:
- Enhances Model Accuracy: Well-annotated images enable the model to learn patterns more effectively, improving overall accuracy.
- Facilitates Object Recognition: Annotation allows models to recognize and differentiate between objects, crucial for applications such as autonomous driving and facial recognition.
- Boosts Multimodal Learning: Integrating image annotation with other data types, such as text, enhances learning processes and produces more comprehensive models.
- Enables Supervised Learning: Most machine learning algorithms require labeled data to learn and make predictions. Image annotation serves as a foundational step in this process.
Types of Image Annotation Techniques
Understanding various image annotation techniques is critical for successful machine learning projects. Here are the most common methods:
1. Image Classification
In image classification, the entire image is labeled with a single tag, indicating the primary subject or category of the image. For example, a picture of a dog would simply be labeled "dog." This method is often used in applications like content moderation and visual search engines.
2. Object Detection
Object detection involves identifying and labeling multiple objects within an image. Each object is typically enclosed within a bounding box, allowing for precise location recognition. This technique is crucial for self-driving cars and surveillance systems.
3. Semantic Segmentation
This advanced technique categorizes every pixel in an image into a class label, facilitating detailed comprehension of the scene. For instance, in a street view, pedestrians, vehicles, and road signs can all be distinctly labeled. Semantic segmentation is particularly useful in healthcare imaging diagnostics and urban planning.
4. Instance Segmentation
Instance segmentation takes semantic segmentation a step further by not just labeling pixels but also differentiating between individual instances of the same object. This method helps in scenarios like counting cars in traffic or identifying distinct instances of a particular animal species in a wildlife study.
Challenges Associated with Image Annotation
While image annotation provides substantial benefits, it also comes with its own set of challenges:
- Subjectivity in Labeling: Different annotators may interpret images differently, leading to inconsistencies.
- Time-Consuming Process: Annotating large datasets can be labor-intensive and time-consuming.
- Quality Control: Ensuring high-quality annotations requires effective management and verification processes.
- High Costs: Outsourcing annotation can incur substantial costs, particularly for large-scale projects.
Why Choose KeyLabs.ai for Your Image Annotation Needs?
Keylabs.ai offers a sophisticated data annotation tool and data annotation platform designed to streamline the image annotation process:
1. Advanced Technology
Utilizing cutting-edge machine learning algorithms, our platform automates many aspects of the annotation process, significantly reducing human labor and time.
2. Scalable Solutions
Whether you are working with a small dataset or need to annotate millions of images, Keylabs.ai scales with your needs, providing flexibility and adaptability.
3. Quality Assurance
We employ rigorous quality control mechanisms and continuous feedback loops during the annotation process to ensure the highest standards of accuracy.
4. Customizable Workflow
Our platform allows clients to customize workflows, enabling teams to manage their projects efficiently and effectively.
How to Get Started with Image Annotation for Machine Learning
Here’s a step-by-step guide to kick-start your image annotation project:
Step 1: Define Your Objectives
Establish clear goals for what you aim to achieve with your machine learning project. Determine what types of annotations are needed based on the application (e.g., image classification, object detection).
Step 2: Collect Your Data
Gather a comprehensive dataset of images that are representative of the problem domain. Ensure that there is enough diversity in your dataset to train a robust model.
Step 3: Choose the Right Annotation Tool
Select a data annotation platform conducive to your requirements. Keylabs.ai offers various features tailored for efficient image annotation.
Step 4: Start Annotating
Engage trained annotators or use semi-automated tools provided by your chosen platform. Monitor the progress, ensuring accuracy at every stage.
Step 5: Review and Validate
Implement a quality check process to review the annotations. This can involve multiple rounds of validation to verify correctness and consistency.
Step 6: Integrate Annotated Data into Your ML Model
Once the images are annotated and validated, integrate them into your machine learning model and begin the training process.
Conclusion
As AI continues to permeate various industries, the demand for high-quality annotated images will only grow. Image annotation is pivotal in unlocking the full potential of machine learning algorithms. Platforms like Keylabs.ai are at the forefront, providing cutting-edge solutions to make image annotation more efficient and accurate.
For anyone looking to embark on a machine learning journey or enhance their existing projects, focusing on quality image annotation is not just advantageous—it’s essential. Embrace the power of image annotation for machine learning and elevate your AI initiatives to new heights.