Understanding Annotation in Machine Learning for Business Applications
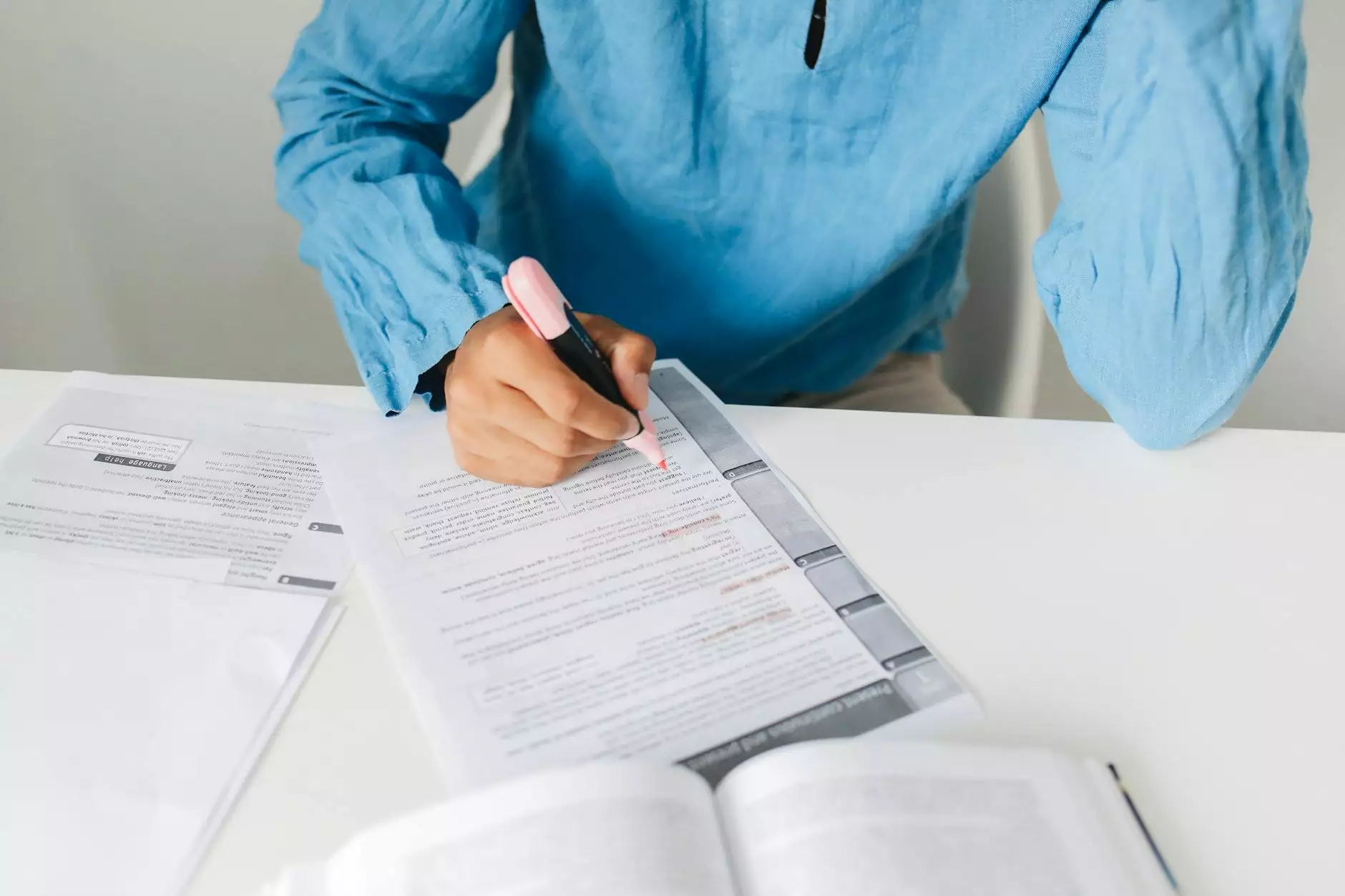
In the fast-paced world of technology, understanding the fundamental concepts behind artificial intelligence (AI) and machine learning has become crucial for businesses aiming to stay competitive. One key component that underpins successful machine learning models is annotation in machine learning. This comprehensive guide will delve into what annotation is, why it matters, and how businesses, especially within the realms of home services and locksmiths, can leverage this process to enhance their operations.
What is Annotation in Machine Learning?
At its core, annotation in machine learning refers to the process of labeling data to provide context. This labeled data serves as training material for machine learning algorithms, allowing them to learn from examples and make predictions or classifications based on new input. Annotations can take various forms, including:
- Image Annotation: Labeling objects within images, useful in computer vision tasks.
- Text Annotation: Marking up text to train natural language processing (NLP) models.
- Audio Annotation: Tagging audio files for speech recognition systems.
- Video Annotation: Identifying objects or events in video footage.
The Importance of Data Annotation in Machine Learning
The significance of annotation in machine learning cannot be overstated. High-quality, accurately annotated data is essential for building robust machine learning models. Here are several reasons why data annotation is critical:
1. Enhances Model Accuracy
A well-annotated dataset enables machine learning models to understand the nuances of the data they are processing. This leads to better predictions and classifications, effectively increasing the overall accuracy of the model.
2. Reduces Bias
Having diverse and comprehensive annotations helps to mitigate bias in AI models. When different scenarios and variations are represented in the training data, the resulting model can better generalize its predictions.
3. Facilitates Feedback Loops
Annotation allows businesses to create feedback mechanisms. By observing the predictions made by the machine learning models, companies can refine their data and improve the annotation process, leading to iterative enhancements in model performance.
How Businesses Can Leverage Annotation for Competitive Advantage
Companies in home services, keys, and locksmiths can harness the power of annotation in machine learning to gain a competitive edge. Here’s how:
1. Customer Support Automation
By annotating customer inquiries and responses, businesses can train chatbots or virtual assistants to handle routine queries efficiently. This frees up human resources for more complex issues, enhancing operational efficiency.
2. Improved Service Recommendations
Using annotated data from previous customer interactions, businesses can predict future customer needs. For instance, if a customer frequently requests key duplication services, an annotated machine learning model can suggest related services like home security assessments.
3. Smart Inventory Management
Annotation can be applied to predict inventory needs based on seasonal demand. By labeling historical sales data, machine learning algorithms can forecast when certain products, such as specific locks or key types, will be in higher demand.
Implementing Annotation in Your Business Workflow
Integrating annotation in machine learning into your business processes may seem daunting, but with a structured approach, it can be effectively managed. Here’s a simple step-by-step process:
Step 1: Identify Objectives
Clearly define what you aim to achieve with machine learning. Whether it's enhancing customer service or revolutionizing inventory management, having a focused goal is essential.
Step 2: Gather Data
Collect data that is relevant to your objectives. This may include customer interactions, sales data, or operational metrics.
Step 3: Annotate the Data
Engage skilled annotators or utilize annotation tools and platforms that can facilitate the labeling process efficiently. Ensure that the annotations are accurate and comprehensive.
Step 4: Train the Machine Learning Model
Using the annotated dataset, train your machine learning model. Test and validate its performance to ensure it meets your business objectives.
Step 5: Evaluate and Iterate
After deployment, continuously monitor the model’s performance. Use feedback loops and new data to refine and improve your annotations and model training.
Challenges in Data Annotation
While annotation is vital, it does come with its challenges. Understanding these can help businesses prepare and devise solutions:
1. Time-Consuming Process
Annotating data can be a lengthy process, especially for large datasets. This may impact project timelines and resource allocation.
2. Requires Expertise
Quality annotations require knowledgeable annotators who understand the business context and data intricacies. Investing in training or hiring skilled professionals can mitigate this.
3. Maintenance of Annotated Data
As business needs evolve, previously annotated data may become outdated. Continuous updating and maintenance of datasets are necessary to remain relevant.
Conclusion
In conclusion, annotation in machine learning is a fundamental element that empowers businesses to harness the potential of AI effectively. By understanding its significance and implementing it strategically, companies in the home services and locksmith sectors can improve their operational efficiency, enhance customer experiences, and ultimately, achieve greater success in a competitive marketplace. Embrace machine learning today and annotate your way to a smarter, more responsive business.
Call to Action
If your business is ready to take the next step into the world of AI and machine learning, consider investing in quality data annotation services. With the right strategies and support, you can transform the way your business operates and deliver exceptional value to your customers.